Transforming Healthcare Economics: The Role of Artificial Intelligence in Revenue Cycle Management
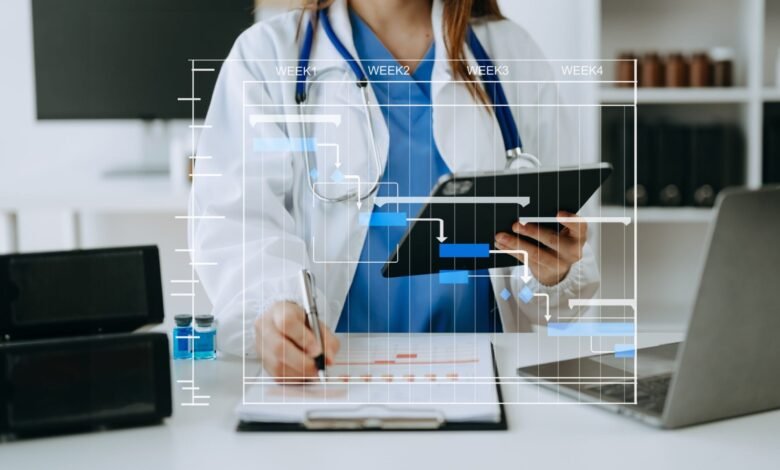
In today’s increasingly complex healthcare environment, managing financial operations has become just as critical as delivering quality patient care. Revenue Cycle Management (RCM), which encompasses the entire financial life cycle of a patient—from scheduling and registration to final payment—plays a pivotal role in a provider’s financial health. However, traditional RCM methods often suffer from inefficiencies, manual errors, and rising operational costs.Artificial Intelligence revenue cycle management Once confined to the realms of science fiction, AI is now reshaping industries—including healthcare. With its ability to analyze vast datasets, automate repetitive tasks, and deliver predictive insights, AI has emerged as a powerful tool for transforming RCM. This article explores how AI is revolutionizing revenue cycle management, the challenges it addresses, its tangible benefits, and what the future holds.
Challenges in Traditional Revenue Cycle Management
Manual Errors and Inefficiencies
Traditional RCM relies heavily on human input for data entry, billing, and coding. This manual approach is prone to errors—misspelled patient information, incorrect codes, or mismatched insurance details—all of which can lead to claim denials and delayed payments.
Delayed Claims and Denials
Healthcare providers frequently face claim rejections due to inaccurate or incomplete information. These delays not only strain cash flow but also require significant resources to reprocess, often resulting in lost revenue.
Lack of Predictive Insights
Conventional systems are reactive, not proactive. Without advanced analytics, providers cannot easily identify which claims are likely to be denied, or which patients are at risk of non-payment, leading to inefficiencies in collection strategies.
Regulatory Pressures
The healthcare industry is tightly regulated. Changes in billing codes, payer requirements, and compliance laws add complexity and increase the likelihood of non-compliance and financial penalties.
AI-Driven Innovations in Revenue Cycle Management
Automated Coding and Documentation
AI, particularly Natural Language Processing (NLP), can analyze physician notes and convert them into standardized medical codes. This not only improves accuracy but also reduces the time required for documentation and billing.
Predictive Analytics for Denial Management
Machine learning models can analyze historical claims data to predict which claims are most likely to be denied and why. Armed with this insight, billing teams can take corrective actions before submission, significantly reducing denial rates.
Intelligent Scheduling and Eligibility Verification
AI-powered systems can verify a patient’s insurance eligibility in real time, identify coverage gaps, and suggest optimal appointment times based on payer policies and patient preferences, reducing missed appointments and surprise denials.
Robotic Process Automation (RPA) in Billing
RPA can mimic human actions to execute rule-based tasks such as submitting claims, following up on unpaid bills, and posting payments. These bots operate around the clock, improving efficiency and consistency.
Key Benefits of AI Integration in RCM
Increased Revenue and Faster Reimbursements
By improving coding accuracy, reducing claim denials, and speeding up processing times, AI accelerates the reimbursement cycle and helps healthcare providers capture more revenue.
Reduced Administrative Burden
Automation handles repetitive tasks, allowing billing teams to focus on more strategic functions such as patient engagement, audit preparation, and process improvement.
Enhanced Accuracy and Compliance
AI tools can detect anomalies, ensure coding is aligned with regulatory standards, and flag non-compliant claims—safeguarding providers from costly penalties.
Improved Patient Financial Experience
AI enhances transparency by offering real-time cost estimates, payment plan options, and personalized billing communications—leading to higher patient satisfaction and better collection rates.
Implementation Strategies and Considerations
Integration with Existing Systems
Seamless integration with Electronic Health Records (EHR) and billing platforms is essential. Interoperability ensures that AI tools can access relevant data to deliver meaningful insights and automation.
Staff Training and Change Management
Introducing AI requires a cultural shift. Training staff to work alongside AI tools, understand their outputs, and trust automated processes is crucial for successful adoption.
Data Privacy and Security
AI systems handle sensitive patient information. Ensuring HIPAA compliance, implementing encryption, and performing regular audits are vital to maintaining data integrity and patient trust.
ROI Evaluation
Measuring return on investment involves tracking improvements in claim acceptance rates, collection times, administrative costs, and overall revenue performance post-AI deployment.
Case Studies and Real-World Examples
Cleveland Clinic: Reducing Claim Denials
Cleveland Clinic implemented an AI-based predictive analytics tool that helped identify and address claim denial triggers. Within a year, denial rates dropped by over 15%, resulting in millions in recovered revenue.
Chatbots in Patient Billing
Several hospitals now use AI chatbots to answer patient billing questions, set up payment plans, and process payments. These virtual agents operate 24/7 and have helped reduce call center volumes by over 30%.
Predictive Financial Risk Models
AI-driven risk scoring models assess a patient’s likelihood of defaulting on payments based on historical behavior and socioeconomic data, allowing providers to offer preemptive financial counseling and payment options.
Future Trends and Opportunities
AI in Value-Based Care
As healthcare shifts from volume to value, AI will play a central role in aligning financial strategies with patient outcomes—helping providers succeed in risk-based contracts.
Interoperability and Decision Support
AI will increasingly serve as a decision-making ally, connecting financial data with clinical outcomes to guide treatment plans, improve documentation, and ensure fair compensation.
Generative AI for Communication
Generative AI tools are being tested for crafting personalized patient statements, financial summaries, and insurance appeals—enhancing clarity and engagement.
Conclusion
Artificial Intelligence revenue cycle management is not just a buzzword—it’s a strategic necessity in today’s healthcare finance landscape. By streamlining operations, minimizing errors, and enabling smarter decisions, AI is redefining how providers manage their revenue cycles. Those who invest in AI today are not only improving their financial stability but also enhancing the overall patient experience.